Re-analysis of hydroxychloroquine and azithromycin trial data
The paper by Gautret and colleagues (1) has generated excitement in the fight against Covid-19. Even President Donald Trump mentioned the study's result. In the paper, Gautret and colleagues conclude that anti-malaria drugs, hydroxychloroquine (HCQ) and azithromycin, are effective in the treatment of patients infected with SARS-Cov-2. However, having analyzed the data in the paper, I have a different interpretation ...
The study is said to have been designed as an open non-randomized trial, but in reality, it was an observational study. The investigators planned to recruit 48 patients, but in the end, 42 patients were included in the study. Actually, the data analysis was based on 36 patients: 20 in the treated group and 16 controls. Treatment was either HCQ or azithromycin -- not randomized. Patients had been followed for 6 days, and during the follow-up, viral load was measured. The rate of negative tests of RT-PCR was the primary outcome. The investiagtors used the Chi-squared test to assess the between-group difference in the rate of negative tests on day 1, 2, 3, ..., 6. Based on the tests, they conclude that:
"Despite its small sample size our survey shows that hydroxychloroquine treatment is significantly associated with viral load reduction/disappearance in COVID-19 patients and its effect is reinforced by azithromycin."
Well, what can we say?
Needless to say, the study design is not optimal. Without a proper randomization, it is very difficult to make a strong inference concerning the effect of the treatment. The attrition was problematic: of the 26 patients in the treated group, 6 were dropped from the analysis because they did not have complete data. More importantly, the analysis was inadequate. Testing for difference between groups at each time point poses two problems: multiplicity of hypothesis testing and within-patient correlation. It is well known that mutiple tests of hypothesis lead to the inflation of type I error, and can result in misleading P-value. The test of hypothesis at each time point implicitly assumes that the serial data were independent, and this is obviously not true. Multiple measurements within a patient are expected to be correlated, and the correlation, if not accounted for, can lead to wrong P-values.
The most appropriate method for this study design is the mixed-effects logistic regression model. In this model, one can elegantly model the change in an outcome (eg negative test) over time for each patient. That is, each patient has a trajectory of change which is represented by a slope. The collection of slopes across patients can then be used for testing the hypothesis of treatment effect. Based on the authors' data (in the paper's appendix), I have conducted a mixed-effects logistic regression analysis. The model is [2]:
log(P / (1 - P)) = Day + HCQ + Azi + HCA x Day + Azi x Day + (nested within patient)
where P is the probability of negative tests. In the above model, the HCA x Day parameter represents the difference in the time-elated slope between patients on HCQ and patients not on HCQ. Similarly, the Azi x Day term represents the interaction effect of azithromycin and time. Result of model fitting can be summarized in the following table:
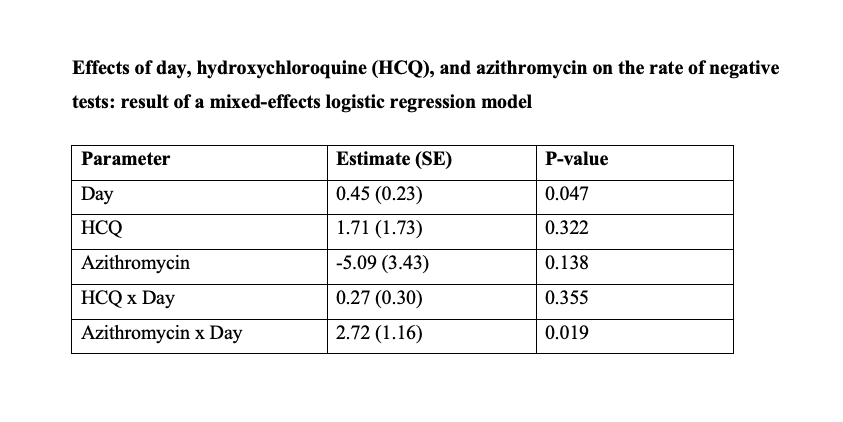
As can be seen from the estimated parameters, the change in the rate of negative tests (hereby referred to as 'outcome') between those on HCQ and those not on HCQ was not statistically significant (P = 0.355). However, patients on azithromycin appeared to have a greater rate of change in the outcome compared with those not on azithromycin (P = 0.019). The changes associated with HCQ and azithromycin can be seen from the following figure which is constructed from the mixed-effects logistic regression model. The figure shows that azithromycin seems to work better than HCQ! However, given the modest sample size, I don't want to place a heavy weight on this observed effect.
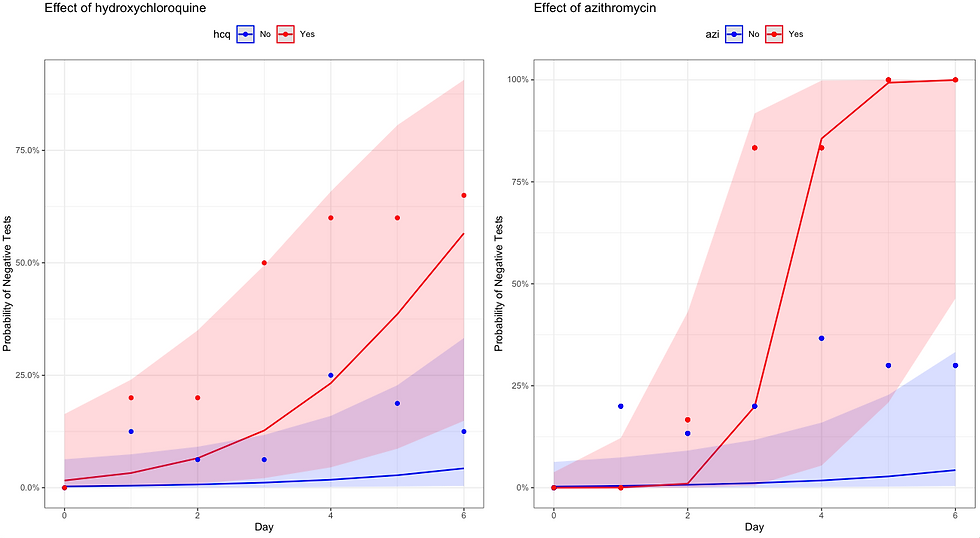
Figure: Predicted probability of negative tests from day 1 to day 6 among patients on HCQ (left panel) and azithromycin (right panel).
So, on the basis of the results presented, I would like to offer an alternative interpretation: the effect of hydroxychloroquine and azithromycin on the elimination of SARS-Cov-2 viral load remains uncertain. I hope that President Trump will consider my interpretation.
Reference
(1) Gautret P, Lagierac JC, Parola P, Hoang TV, Meddeb L, Mailhe M, Doudier B, Courjon J, Giordanengo V, Vieira VE, Dupont HT, Honoré S, Colson P, Chabrière E, La Scola B, Rolain JM, Brouqui P, Raoult D. Hydroxychloroquine and azithromycin as a treatment of COVID-19: results of an open-label non-randomized clinical trial. International Journal of Antimicrobial Agents, 20 March 2020, 105949
[2] In 'lme4' language this model is: glmer(outcome ~ hcq + azi + time + hcq:time + azi:time + (1 | id), data=df, family = binomial(link="logit"))
Em cam on thay !