The blind faith in the P-values should be stopped
- tuan nguyen
- Mar 20, 2019
- 5 min read
The blind faith in the P-values has done innumerable damage to science for ~100 years. It is now high time to stop the dichotomization of significant vs non-significant findings. I am a signatory to the call for stopping the “dichotomia” [1].
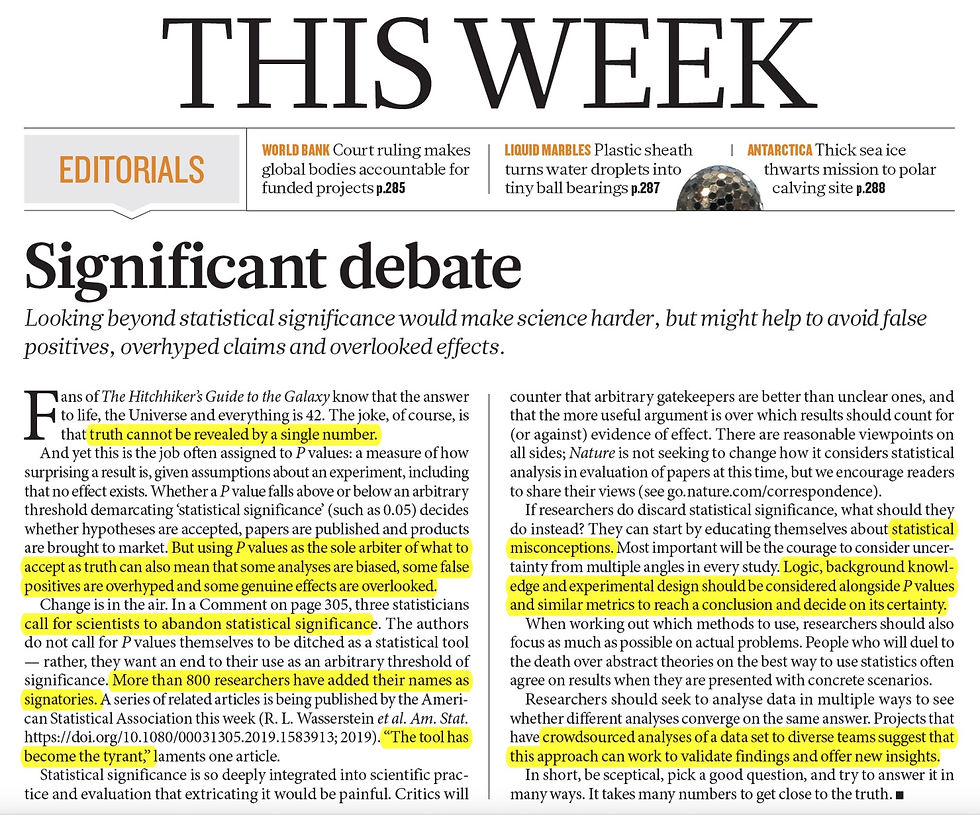
P-value is an invention of the eminent British statistician Sir Ronald A. Fisher. In the 1920s, Fisher advanced the idea of “test of significance” to appraise the merit of a scientific hypothesis. The idea is simple and can be described by the following three steps:
(1) set up a null hypothesis [of no effect];
(2) conduct an experiment to collect data relevant to the hypothesis; and
(3) compute the significance probability of obtaining the data at least as extreme as the ones obtained if the null hypothesis is true.
That probability is called the P-value, or "significance probability" in Fisher's language. Fisher viewed the P-value as an index of strength of evidence against the null hypothesis, and he suggested to use the threshold of 0.05 as a criterion to judge whether a finding is "significant" or not. He advised scientists to "take 5% as a standard level of significance, in the sense that they are prepared to ignore all results which fail to reach this standard" (Design of Experiments 1935, p.13). Fisher urged scientists to publish the exact level of significance, e.g., P = 0.05, not P < 0.05.
However, test of significance has been severely criticized ever since its introduction. The distinguished Polish mathematician Jerzy Neyman commented that Fisher's test of significance was "mathematically specifiable sense, worse than useless" [2]. In 1928, Neyman and Egon Pearson (a son of Karl Pearson, the inventor of Chi square statistic) argued that Fisher's method was illogical, because one could not consider a null hypothesis without conceiving one plausible alternative hypothesis. Neyman and Pearson then developed the theory of test of hypothesis, which can be summarized as follows: (1) set up a null hypothesis and an alternative hypothesis of interest; (2) determine the value of α (the probability of falsely rejecting the null hypothesis) and 1-β (the probability of correctly rejecting the null hypothesis); the two values define a "critical region" for a summary statistic; (3) collect data relevant to the hypotheses, and compute the statistic; (4) make decision: if the statistic fell within the critical region, then the alternative hypothesis is accepted; if not, the null hypothesis is accepted. The test of hypothesis advocated by Neyman-Pearson was designed so that "in the long run of experience, we shall not be too often wrong" (On the Problem of the Most Efficient Tests of Statistical Hypotheses, 1933).
Note that the Fisher's test of significance can only reject or accept the null hypothesis, but the Neyman-Pearson's test of hypothesis allows scientist to make decision concerning both null and alternative hypothesis. For Fisher, the P-value is a property of the data, but for Neyman and Pearson, α and β are properties of a statistical test. Fisher was not impressed with the Neyman-Pearson's test of hypothesis, and he had become a vociferous critic of the test for 27 years (until Fisher's death in 1962). According to L. J. Savage, Fisher "published insults that only a saint could entirely forgive" [3]. Most of the debates between Fisher and Neyman and Pearson were waged on philosophical grounds, but Fisher made an important point that the test of hypothesis uses of acceptance or rejection based on models formulated before data are collected was not compatible with practical situations faced by scientists.
Notwithstanding the disagreement between those founders, the predominant model of inference nowadays is am amalgamation of test of significance and test of hypothesis, which neither Fisher nor Neyman and Pearson would approve! The new "hybrid model" has become a quasi gold standard in experimental science. This hybrid model can be summarized by the following 4 steps:
· State the null hypothesis and an alternative hypothesis;
· Determine the α and β levels, and sample size;
· Collect the data, and compute the P value;
· If P value < α, accept the alternative hypothesis; if not, accept the null hypothesis.
The P value used in this hybrid model does not have the same meaning as Fisher's intent.
According to the new hybrid model of inference, any finding with P-value < α (where α is isually set at 0.05) is considered “significant”. By consequence, any finding with P-value > α (say 0.051) is regarded as “not significant”. Of course, such a dichotomization is absurd, because the P-value can vary from sample to sample [4]. The sharp distinction between P = 0.049 and P = 0.051 is clearly not justifiable. Yet, in practice that distinction is practised by many scientists. That leads to questionable practice of “P-hacking” [5] and data dredging [6]. All of those practices have contributed to the crisis of irreproducibility in scientific research, and have done enormous damage to science in general.

“If all else fails, use ‘significant at P > 0.05 level’ and hope no one notices.” (http://xkcd.com/1478/, Randall Munroe, Creative Commons Attribution-NonCommercial 2.5 License)
In 2016, the American Statistical Association (ASA) issued a “Statement on Statistical Significance and P-value” [7] calling for a reasoned use of P-value. The Statement clearly advises that “P-value was never intended to be a substitute for scientific reasoning,” any interpretation of finding must be considered within context. The Statement advances 6 principles for the proper use of significance testing and P-values:
· P-values can indicate how incompatible the data are with a specified statistical model.
· P-values do not measure the probability that the studied hypothesis is true, or the probability that the data were produced by random chance alone.
· Scientific conclusions and business or policy decisions should not be based only on whether a p-value passes a specific threshold.
· Proper inference requires full reporting and transparency.
· A p-value, or statistical significance, does not measure the size of an effect or the importance of a result.
· By itself, a p-value does not provide a good measure of evidence regarding a model or hypothesis.
Although the ASA Statement has attracted a lot of attention from popular media as well as scientific media, the practice of dichotomization of P-value is still widespread. It is hoped that with the publication of this piece [1] in Nature, the scientific community will take note of the reasoned use of P-value and signficance test.
So, what is the “reasoned use” – I hear you ask. While this note is not about that question, I propose the following:
· Be clear about the hypothesis that you want to test;
· Make use of confidence interval;
· Pay attention to practical/clinical significance rather than “statistical significance”;
· Resort to Bayesian inference;
· Make use of Bayes Factor more often in your research.
====
[2] Gigerenzer G, Swijtink Z, Porter T, Daston L, Beatty J, Kruger L. The Empire of Chance: How Probability Changed Science and Everyday Life. Cambridge University Press. 1989;London
[3] Savage L. On rereading A. A. Fisher. Annals of Statistics. 1976;4(3):441-500.
Cám ơn Giáo sư rất nhiều. Tôi ước gì Giáo sư có một bài phân tích và đánh giá khách quan về các kết quả phân tích tổng hợp (meta-analysis) gần đây được công bố rất nhiều trên các tạp chí y dược quốc tế. Mặc dù tin tưởng vào toán học, tôi luôn phân vân về các kết quả rút ra từ các tính toán ấy vì nó gần như phủ nhận hầu hết các kết quả lâm sàng dương tính và kinh nghiệm cổ truyền của các cây thuốc và bài thuốc dân gian cũng như của các hoạt chất chiết xuất được từ đấy.